AI is taking over the business world like never before and in a good way. While AGI may still be a few years away, most businesses are embracing this new intelligence to make their processes faster, effective and efficient.
From creating simple email templates to predicting the next best move in the stock market, AI has multiple use cases.
For all those aspiring entrepreneurs who want to be part of this revolution, here are 10 profitable AI business ideas that you can implement ASAP.
Profitable AI Business Ideas
1. AI Agent for Competitor API Monitoring and Cost Tracking
AI is just the perfect tool to automate API monitoring, track competitor pricing, and provide real-time cost optimization insights.
How AI Helps:
- Automated API Monitoring
- AI-powered crawlers scan competitor APIs, detecting changes in endpoints, pricing, and feature updates.
- Anomaly detection identifies unexpected price shifts, new feature additions, or API restrictions.
- AI tracks API availability, response times, and rate limits to assess service reliability.
- Cost Tracking & Optimization
- AI analyzes past API pricing trends to predict future cost fluctuations.
- Aggregates real-time pricing data from multiple API providers for cost-effective decision-making.
- Sets up automated alerts when competitors adjust pricing or introduce new models.
- Competitive Analysis & Benchmarking
- AI scans forums, reviews, and social media to analyze how users perceive competitor APIs.
- Compares API documentation to identify missing or additional features in rival offerings.
- Tracks update frequency to predict competitor strategies.
- Decision-Making & Forecasting
- AI-powered dashboards visualize competitor movements for quick analysis.
- Predicts market trends based on competitor API changes.
- Recommends strategic adjustments like pricing tweaks or feature enhancements.
Industry Example:
o Kompyte: Kompyte uses AI to gather and analyze competitor data across online sources. It provides real-time insights of the competition, allowing sales, marketing, and product teams to make decisions based on competitor activity, like pricing changes, new product launches, and market trends. It automates the process of competitive intelligence gathering.
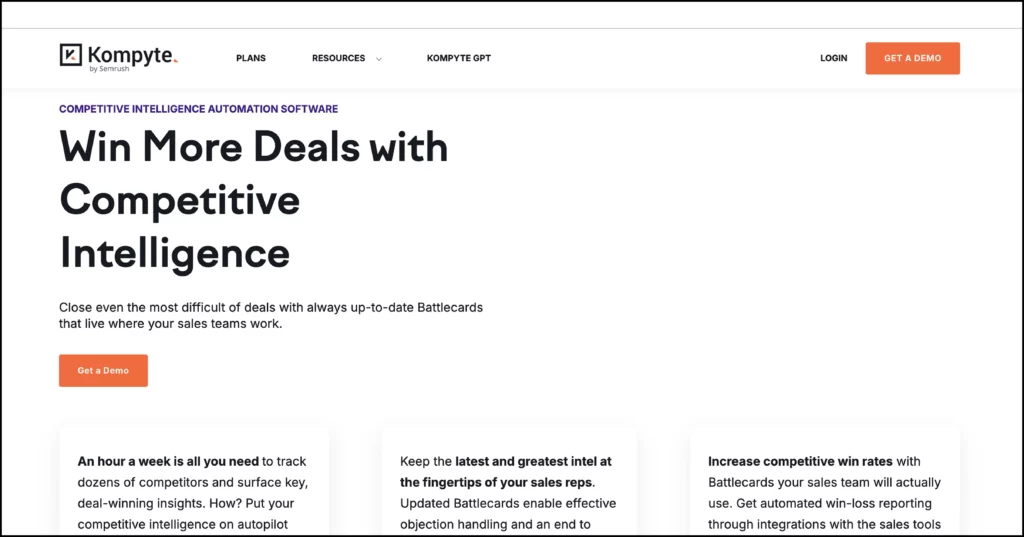
Applications:
- Businesses track competitor strategies using AI to stay competitive.
- Actionable insights for pricing and product development.
Revenue Model: Subscription-based pricing / through licensing.
2. AI-Powered Industry-Specific Datasets Marketplace
AI can identify high-potential GitHub repositories by evaluating code quality, engagement, and commercial potential.
How AI Helps:
- Code & Project Evaluation
- AI scans repositories for best practices, security vulnerabilities, and maintainability.
- Uses NLP models to assess documentation completeness and clarity.
- Contributor & Activity Analysis
- Tracks commit frequency, issue resolution speed, and contributor engagement.
- Identifies trending repositories based on forks, stars, and pull requests.
- Market & Commercial Viability
- AI compares repository functionality with market needs to determine product-market fit.
- Predicts repository growth and potential monetization opportunities.
- Acquisition & Valuation Insights
- AI estimates acquisition costs by analyzing licensing models, user adoption, and similar past transactions.
- Provides competitor intelligence by tracking who forks or watches specific repositories.
Industry Example:
o Kaggle Datasets: The Kaggle platform allows users to discover, access, share, and utilize a huge collection of public datasets. These have various applications like data science projects. While you too can create and upload your own custom datasets. This central hub is useful for building and training ML models.
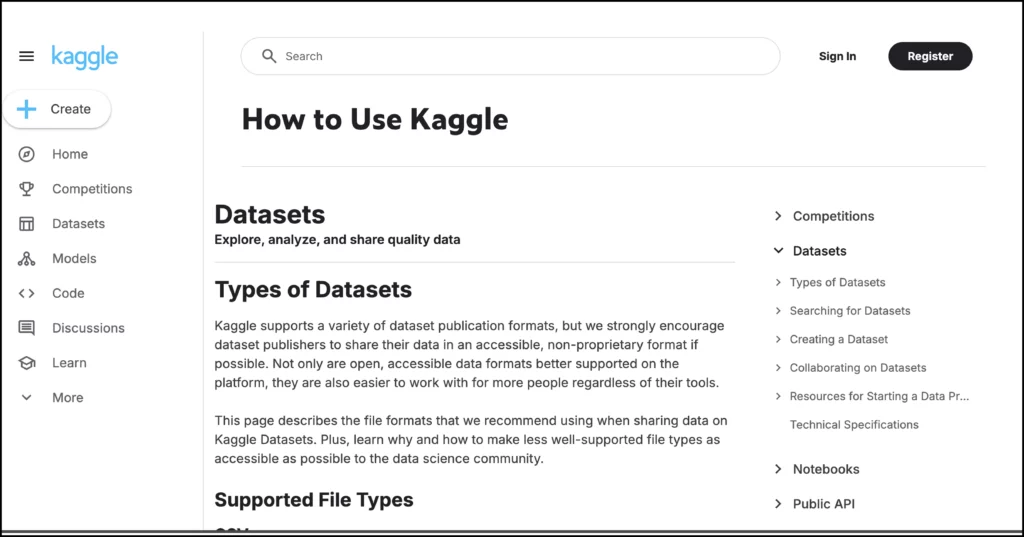
Applications:
o Data scientists and AI developers use these datasets to build and train models.
o Serves industries like healthcare, retail, and finance.
Revenue Model: Platform fees: Maximum 30% of dataset sales.
3. AI Monitoring AI Model Drift and Performance Over Time
AI ensures machine learning models stay accurate by detecting performance degradation and triggering retraining.
How AI Helps:
- Drift Detection & Monitoring
- AI continuously analyzes incoming data for distribution shifts (concept drift, data drift).
- Detects feature importance changes, helping maintain model reliability.
- Automated Model Retraining
- AI triggers retraining workflows when accuracy drops below a threshold.
- Dynamically adjusts hyperparameters to improve model performance.
- Performance Benchmarking
- AI compares predictions with real-world outcomes to measure accuracy.
- Tracks model bias and fairness to ensure ethical AI usage.
- Adaptive Learning Pipelines
- AI integrates with MLOps workflows to automate dataset updates and model retraining.
- Suggests additional training data when performance degradation is detected.
Industry Examples:
o Arize AI: This ML observability platform helps monitor and analyse the performance of a deployed AI model in real-time, to detect issues, troubleshoot root causes, and improve overall model performance.
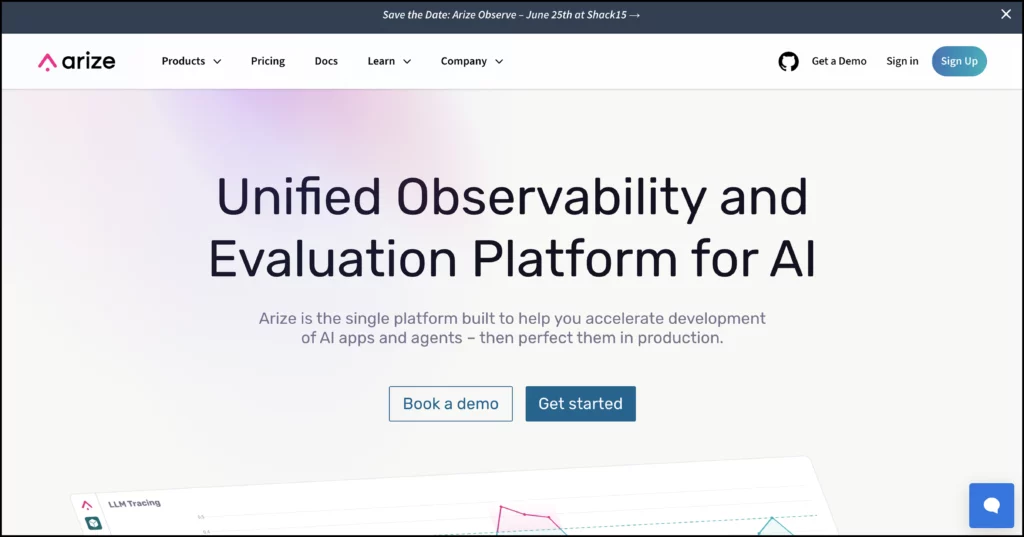
o Fiddler AI: This ‘AI observability platform’ focuses on monitoring, analysing, and protecting ML models in production to ensure their AI applications are performing well, are true, and comply with AI norms. It helps businesses understand “why” their AI models are making certain predictions and how to take corrective actions when necessary.
Applications:
o Companies use AI to ensure their models remain accurate and reliable over time.
o Critical for industries like finance, healthcare, and e-commerce.
Revenue Model: Subscription-based pricing / pay-per-use.
4. AI Analysing GitHub Repositories for Acquisition Opportunities
AI enhances data quality, automates curation, and personalizes dataset discovery in a marketplace for industry-specific data.
How AI Helps:
- Data Quality & Verification
- AI assesses dataset completeness, consistency, and accuracy before listing.
- Detects biases and missing values, ensuring high-quality data.
- Automated Data Labeling & Structuring
- AI tags and categorizes raw datasets for easy searchability.
- Enhances unstructured data with automated metadata generation.
- Personalized Search & Recommendations
- AI recommends datasets based on user behavior, past purchases, and project needs.
- Uses NLP to improve dataset search accuracy with context-aware results.
- Pricing & Demand Prediction
- AI forecasts dataset demand and suggests optimal pricing.
- Uses competitor analysis to position datasets competitively in the marketplace.
- Synthetic Data Generation
- AI creates synthetic datasets to supplement real-world data while maintaining privacy.
- Helps industries lacking sufficient real-world data for AI model training.
Industry Examples:
o Sourcegraph: Sourcegraph allows developers to search and navigate through their entire codebase, across multiple repositories, via a search engine that understands code to instantly come up with necessary information and fathom complex systems that exist within their projects.
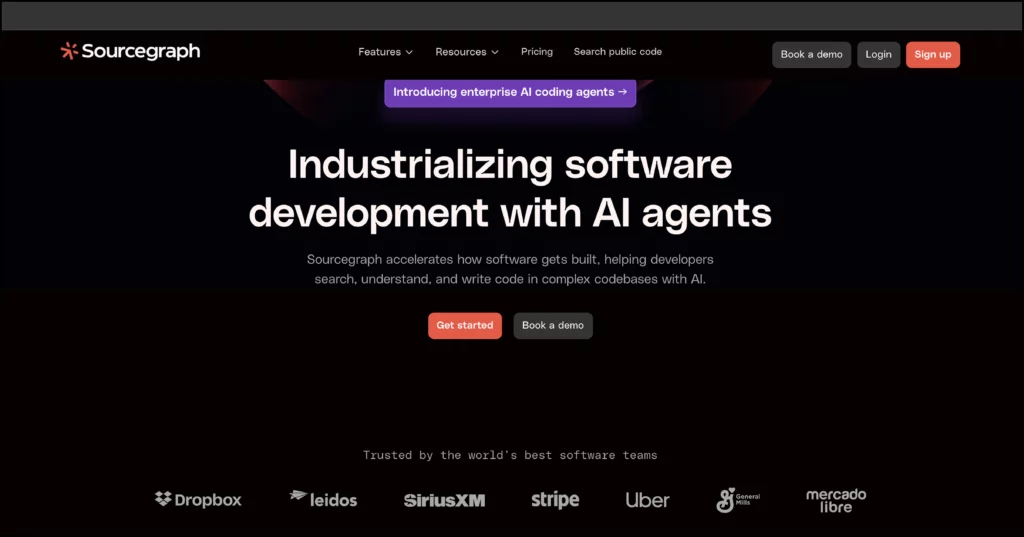
o GitHub’s Advanced Security: A GitHub Advanced Security license provides private repositories features such as code scanning (search for vulnerabilities and coding errors), CodeQL CLI (generates code scanning results that can be uploaded), secret scanning – Detect secrets, (detects secrets being pushed to your repository), custom auto-triage rules (control over your alerts) and dependency review.
Applications:
o AI helps to locate projects or startups that may be undervalued which is of use to investors and acquirers.
o Analyzing GitHub repositories provides insights like user behaviour, what makes a repository popular, technologies trending among developers and trends in open-source development.
Revenue Model: Subscription-based pricing or licensing fees.
5. AI-Powered Real-Time Threat Detection and Response Systems
AI is used to analyze vast amounts of network traffic, detect anomalies, and identify potential security threats in real-time.
- Machine Learning Models: AI learns from past attack patterns to predict and prevent new threats.
- Behavioral Analytics: AI establishes baselines of normal activity and flags deviations.
- Automated Response: AI-powered systems can instantly isolate compromised systems or alert security teams.
Industry Examples:
o Darktrace: This cybersecurity company utilizes AI to monitor and protect your digital environment by learning what’s normal. Hence it can detect any unusual activity and overcome possible cyber threats. Darktrace can autonomously defend against new-age cyber threats like cloud-based threats, ransomware, and insider attacks, that are usually missed by traditional security systems.
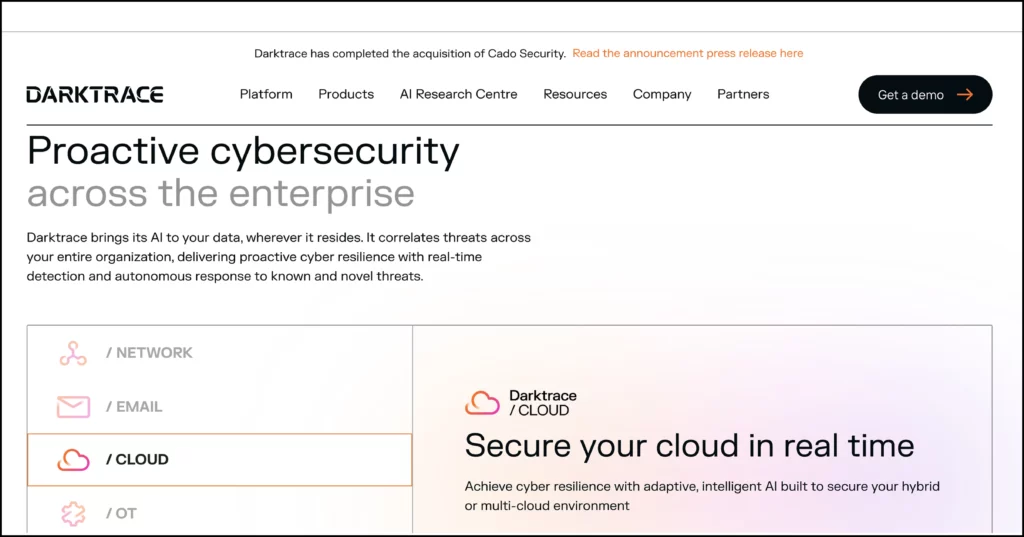
o CrowdStrike: CrowdStrike provides cyber-attack response services, client-side security solutions, and threat intelligence. Their AI driven all-in-one platform helps organizations detect and prevent attacks by monitoring their devices for malicious activity through a cloud-based system.
Applications:
o AI helps organisations prevent insider threats, data incursions, and ransomware attacks.
o Cybercrimes are attended without much delay.
Revenue Model: Subscription-based pricing / licensing fees.
6. AI Detecting Wasted Compute Power Across Cloud Providers
AI helps optimize cloud costs by identifying underutilized resources across AWS, Azure, and Google Cloud.
- Resource Monitoring: AI tracks CPU, memory, and storage utilization.
- Predictive Optimization: AI suggests ways to reallocate or shut down underused instances.
- Automated Cost Savings: AI tools can automatically scale down or terminate inefficient cloud workloads.
Industry Example:
o Spot by NetApp (formerly Spot.io): Uses AI to optimize cloud infrastructure and reduce costs.
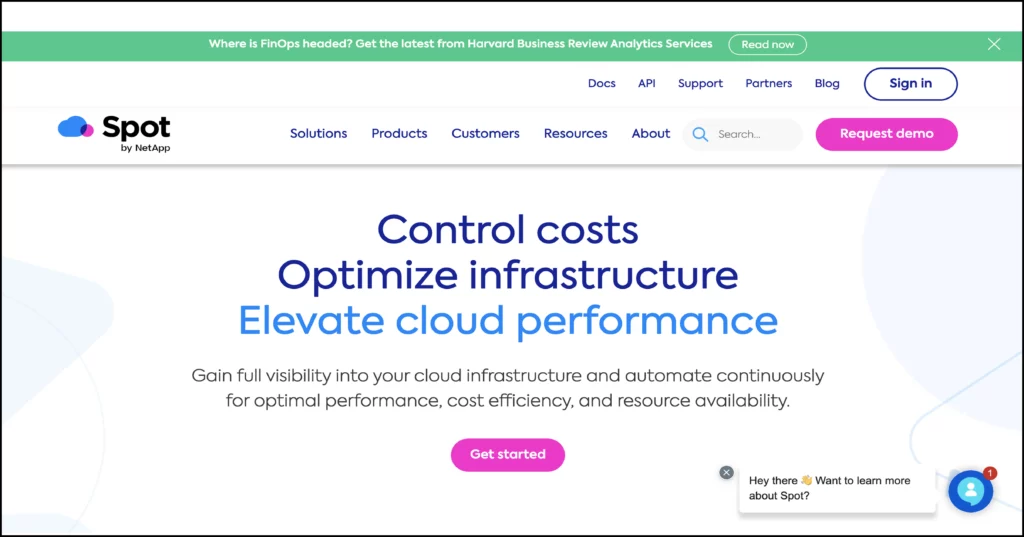
o ParkMyCloud: This cloud cost optimization platform helps identify and eliminate wasted cloud spending automatically across multiple public clouds (like AWS, Azure, and Google Cloud) by scheduling when to “park” (turn off) unused cloud resources.
Applications:
o AI helps cut cloud spending by 30–40%.
o Using these applications startups and SMBs can control cloud budgets efficiently.
Revenue Model: Subscription-based pricing / a percentage of what you save.
7. AI Finding Duplicate AI Spending Across Enterprise Departments
AI helps enterprises eliminate redundant AI investments across different teams.
- Expense Analysis: AI scans purchase orders, invoices, and contracts for duplicate AI tools or services.
- Cross-Department Insights: AI detects multiple teams using similar AI solutions without coordination.
- Spend Optimization: AI suggests consolidation strategies to avoid duplication and reduce costs.
Industry Examples:
o Zylo: Zylo helps businesses manage and optimize their SaaS applications via a centralized view of all SaaS subscriptions, tracking license usage, identifying redundant software, and streamlining renewal processes.
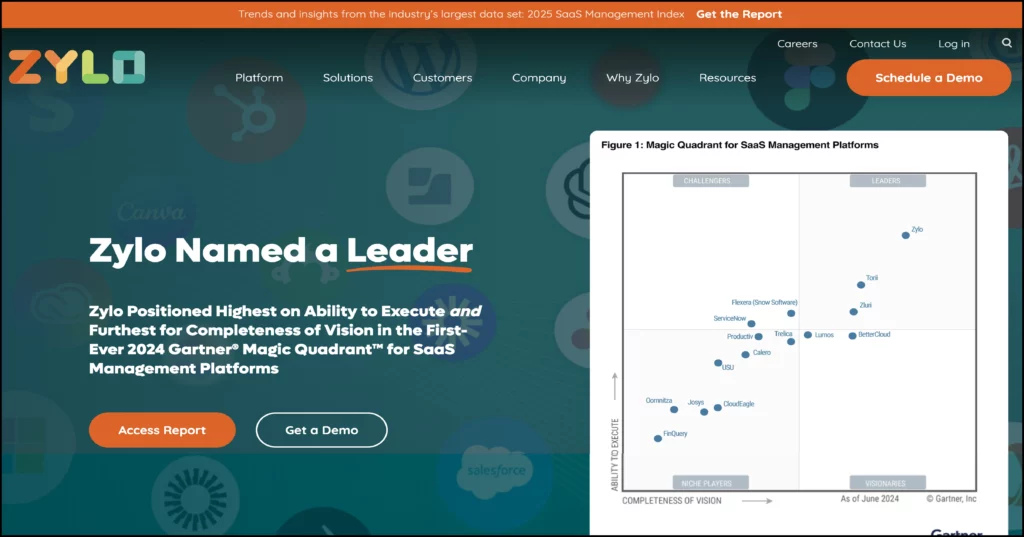
o Torii: Torii helps manage the entire SaaS ecosystem via tools to discover all used applications, automate repetitive tasks, optimize spending, and gain visibility into their SaaS stack.
Applications:
o AI helps merge tools and reduce unnecessary spending.
o Improves ROI on AI investments.
Revenue Model: Licensing fees / percentage of how much you save.
8. AI Detecting Enterprise AI Budgets and Buying Cycles for Sales Teams
AI helps sales teams predict when enterprises are ready to invest in AI solutions.
- Market Intelligence: AI scans public data, job postings, and earnings reports for AI-related signals.
- Predictive Buying Signals: AI identifies companies likely to increase AI budgets.
- Sales Prioritization: AI scores prospects based on readiness to buy, helping sales teams focus on high-value leads.
Industry Example:
o 6sense: This AI-powered company enables businesses to identify and engage with possible buyers. The best accounts can then be targeted by knowing the characteristics of the company (firmographic) and target market (demographic).
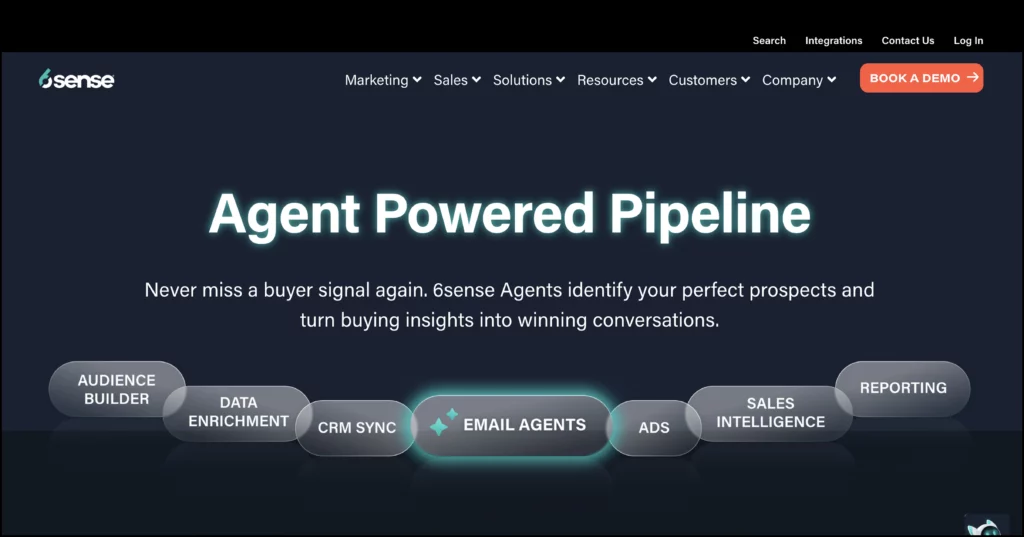
Applications:
o AI helps sales teams reach out to prospects and target high-value customers.
o With the help of AI, companies can now cut the time spent on lead generation and even help land lucrative deals.
Revenue Model: Subscription-based pricing / pay-per-lead.
9. AI Automates Complex API Integrations for SaaS Tools
AI simplifies and accelerates the process of integrating different software tools by automating API interactions.
- AI-Driven Mapping: AI understands API structures and auto-generates integration workflows.
- Code-Free Automation: AI creates API calls and data transformations without requiring manual coding.
- Self-Healing APIs: AI detects API changes (e.g., version updates, deprecations) and adjusts integrations automatically.
Industry Examples:
o Zapier: This web automation tool connects web apps and services. It automates and streamlines your online workflows without coding. With Zapier you can automate workflows on social media, project management, note-taking, email, or meetings.
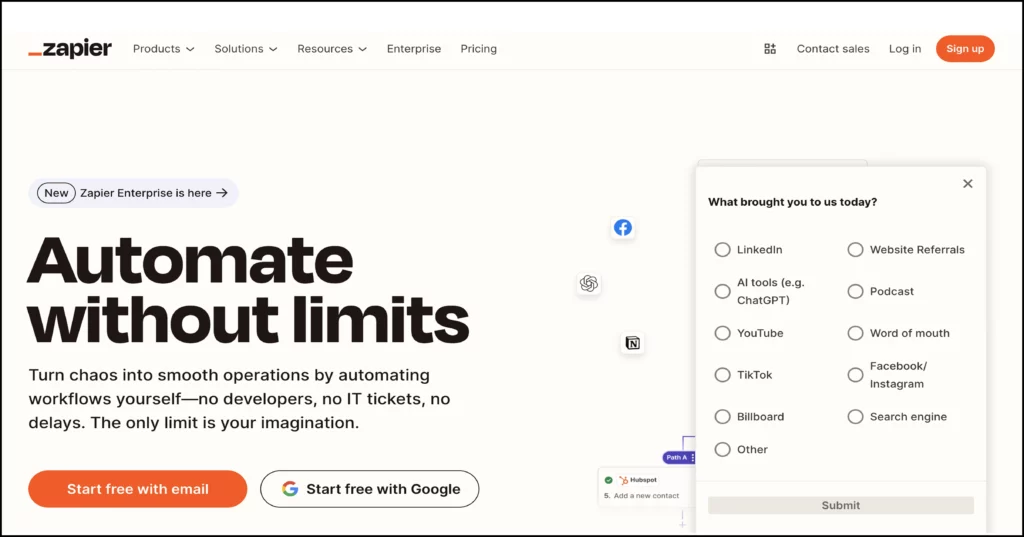
o Make (formerly Integromat): Make is a visual automation platform to design and build complex workflows by connecting different applications and services without coding. They streamline repetitive tasks across various software via automated processes through a drag-and-drop interface. They also enable no-code automation for API integrations.
Applications:
o AI is used to connect tools like CRM, marketing automation, and analytics.
o On the whole businesses improve their operational efficiency and reduce manual work.
Revenue Model: Subscription-based pricing / pay-per-integration.
10. AI Detecting Duplicate Workflows Across Different Tools (Notion, Asana, Trello)
AI identifies redundant or overlapping workflows across multiple productivity tools to improve efficiency.
- Data Synchronization Analysis: AI scans task structures, tags, and dependencies across different tools.
- Pattern Recognition: AI detects similar task lists, project templates, or workflow automations across platforms.
- Smart Recommendations: AI suggests merging or streamlining duplicate workflows to prevent inefficiencies.
Industry Example:
- Unito: Unito allows users to easily sync data between different apps and platforms they use for work. Basically it syncs workflows across tools like Asana, Trello, and Notion.
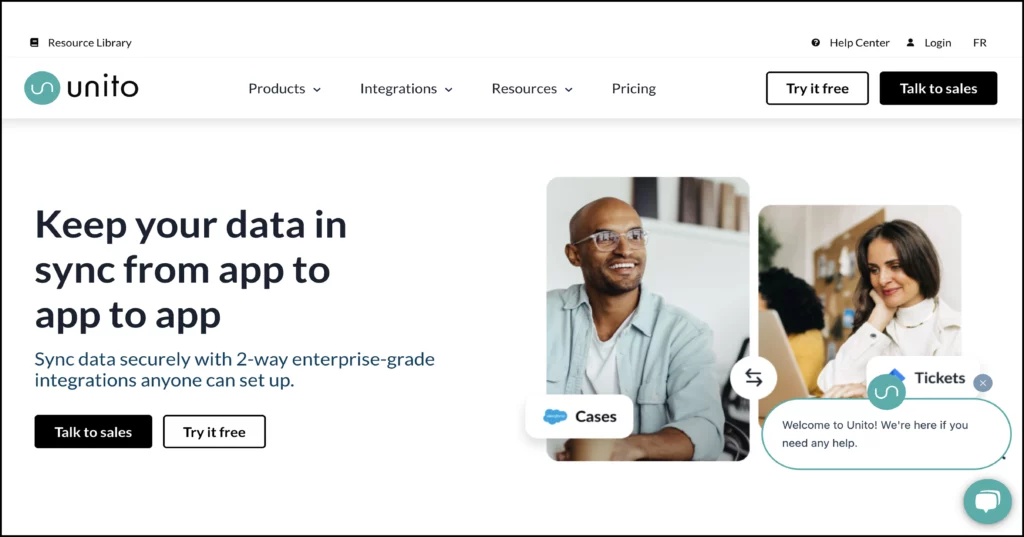
Applications:
- AI helps organise workflows and cut inefficiencies.
- Businesses can optimize resource allocation and task management.
- Revenue Model: Subscription-based pricing / enterprise licensing.
Challenges for AI-Based Businesses & How to Overcome Them
There isn’t any doubt that AI-based businesses offer huge potential for growth and revenue generation, but these are not without challenges. To grow a sustainable AI business, it is imperative to come in terms with technical, legal, and market barriers. Also focus on accessibility, compliance, and differentiation to find success.
Let’s take up the key challenges and practical ways to overcome them.
Challenges & Solutions:
Challenge | Solution |
Technical Barriers & Costs | Use low-code/no-code platforms, outsource development, leverage cloud tools. |
Data Privacy & Compliance | Adopt privacy-by-design, ensure regulatory compliance, and mitigate bias. |
Scalability & Adaptability | Build modular systems, implement continuous learning, and integrate APIs. |
Talent Acquisition & Retention | Upskill teams, offer remote work, and provide equity or incentives. |
How to Check If an AI Business Idea Is Practical
To find if an AI business idea is practical, begin by evaluating the market demand, technical feasibility, and financial sustainability.
The chances of success can be worked out through ROI analysis and a structured framework as shown below.
1. Framework for Evaluating AI Business Idea Feasibility
The feasibility of an AI business idea can be assessed by a structured approach as shown. Below is a comprehensive framework:
A. Market Validation
· Target Audience: The first step to launch a product is to identify your target audience, that is those who will use the product and benefit from it.
· Market Demand: A product finds acceptance if it solves a pain point. So find the pain point you plan to solve.
· Competitive Landscape: Knowing the competition is key, that is if there are other companies also in the same niche. Then identify gaps in their service and in the market.
· Regulatory & Ethical Considerations: Compliance with AI regulations and ethical AI principles is utmost imperative.
B. Technological Feasibility
· AI Model Viability: Determining the feasibility of a project before beginning development is vital.
· Data Availability: Assess the availability of copious amounts of high-quality data.
· Infrastructure & Scalability: Keep in mind the scalability and computational needs of the AI solution.
C. Business Model Viability
· Revenue Streams: Identify various monetization strategies that can be adopted like subscription, licensing, SaaS, etc.
· Cost Structure: Estimate development, maintenance, and operational costs.
· Scalability & Expansion: Analyse if the business model supports growth and expansion.
2. ROI Analysis and Revenue Projections
For financial feasibility, performing ROI analysis is crucial.
A. Cost Estimation
· Development Costs: Hiring staff, AI model training, acquiring datasets, and infrastructure costs need to be taken into account.
· Operational Costs: Cloud computing, maintenance, customer support, and marketing expenses come under operational costs.
· Compliance & Security: Investing in regulatory compliance and cybersecurity is also vital.
B. Revenue Projection Models
· Subscription Model: This model provides recurring revenue from users subscribing to the service.
· Transaction-based Model: Revenue generated per use or transaction.
· Enterprise Licensing: Charging businesses a licensing fee for using the software.
· Advertising & Data Monetization: Utilising AI-driven insights for targeted advertising.
C. ROI Calculation
ROI Calculation evaluates profitability and financial viability using key financial metrics like Customer Acquisition Cost (CAC), Lifetime Value (LTV), Gross Margin, and Break-even Analysis to evaluate profitability.
Compare CAC and LTV to ensure customer acquisition costs are justified by long-term value.
Implement sensitivity analysis to understand different financial scenarios by adjusting variables (e.g., pricing, costs, market conditions). Utilizing best financial planning software can streamline these calculations, automate data analysis, and provide deeper insights into optimizing financial strategies.
FAQs
1. What is the best AI business to start?
AI Agent for Competitor API Monitoring
AI-Powered Datasets Marketplace
AI for Monitoring Model Drift
AI Analyzing GitHub Repositories
AI-Powered Threat Detection
AI for Cloud Cost Optimization
AI for Eliminating Duplicate AI Spending
AI for Sales Intelligence
AI Automating API Integrations
AI Detecting Duplicate Workflows Across Tools
2. Do I need to be a programmer or AI expert to start an AI business?
No, you can build a team with the necessary technical expertise while focusing on the business strategy, market understanding, and identifying problems that AI can solve within your chosen industry.
3. What are some underrated AI business ideas with high potential?
There are many lesser-known but promising ideas like AI-powered culinary personalization apps, AI-driven fraud detection for niche industries, AI-based content authenticity checkers, AI-powered energy optimization solutions, AI-assisted legal contract review, AI-generated interior design, and AI for automated podcast editing and transcription.
4. How long does it take to develop and launch an AI startup?
The timeline to develop and launch an AI startup varies on several factors like the complexity of the project. Even though a simple AI-powered chatbot can be launched in weeks, a fully AI-driven SaaS platform could take anything from 6-12 months.
5. What are the biggest risks of running an AI-based business?
The biggest risks running an AI business include data privacy concerns, regulatory changes, and model in
accuracies that can affect decision-making. Regular audits and legal compliance help mitigate these risks.
6. How do I start my own AI business?
To launch an AI business, define a niche (e.g., cybersecurity, automation, SaaS). You need basic AI knowledge, technical or entrepreneurial experience, and $10K–$100K+ for MVP development. Operate in tech hubs (USA, EU, Asia). Leverage cloud AI tools, secure funding (bootstrapping, VC), and validate demand via pilots before scaling.